10: Audio and Visual Displays [Q&A Session]
-
Full Access
-
Onsite Student Access
-
Virtual Full Access
Date/Time: 06 – 17 December 2021
All presentations are available in the virtual platform on-demand.
Binaural Audio Generation via Multi-task Learning
Abstract: We present a learning-based approach to generate binaural audio from mono audio using multi-task learning. Our formulation leverages additional information from two related tasks: the binaural audio generation task and the flipped audio classification task. Our learning model extracts spatialization features from the visual and audio input, predicts the left and right audio channels, and judges whether the left and right channels are flipped. First, we extract visual features using ResNet from the video frames. Next, we perform binaural audio generation and flipped audio classification using separate subnetworks based on visual features. Our learning method optimizes the overall loss based on the weighted sum of the losses of the two tasks. We train and evaluate our model on the FAIR-Play dataset and the YouTube-ASMR dataset. We perform quantitative and qualitative evaluations to demonstrate the benefits of our approach over prior techniques.
Author(s)/Presenter(s):
Sijia Li, Tianjin University, China
Shiguang Liu, Tianjin University, China
Dinesh Manocha, University of Maryland College Park, United States of America
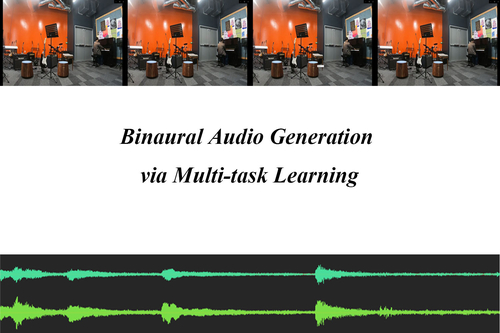
Neural 3D Holography: Learning Accurate Wave Propagation Models for 3D Holographic Virtual and Augmented Reality Displays
Abstract: Holographic near-eye displays promise unprecedented capabilities for virtual and augmented reality (VR/AR) systems. The image quality achieved by current holographic displays, however, is limited by the wave propagation models used to simulate the physical optics. We propose a neural-network-parameterized plane-to-multiplane wave propagation model that closes the gap between physics and simulation. Our model is automatically trained using camera feedback and it outperforms related techniques in 2D plane-to-plane settings by a large margin. Moreover, it is the first network-parameterized model to naturally extend to 3D settings, enabling high-quality 3D computer-generated holography using a novel phase regularization strategy of the complex-valued wave field. The efficacy of our approach is demonstrated through extensive experimental evaluation with both VR and optical see-through AR display prototypes.
Author(s)/Presenter(s):
Suyeon Choi, Stanford University, United States of America
Manu Gopakumar, Stanford University, United States of America
Yifan Peng, Stanford University, United States of America
Jonghyun Kim, NVIDIA Research, Stanford University, United States of America
Gordon Wetzstein, Stanford University, United States of America
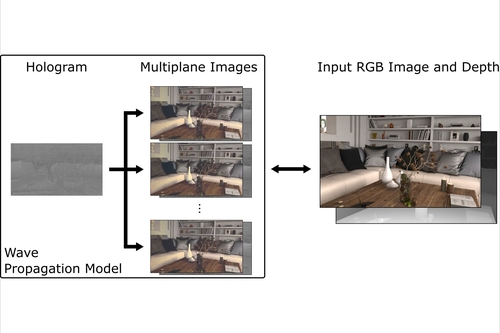
Project Starline: A high-fidelity telepresence system
Abstract: We present a real-time bidirectional communication system that lets two distant users experience a conversation as if they were copresent. It is the first telepresence system that is demonstrably better than 2D videoconferencing, as measured using participant ratings (e.g., presence, attentiveness, reaction-gauging, engagement), meeting recall, and observed nonverbal behaviors (e.g., head nods, eyebrow movements). This milestone is reached by maximizing audiovisual fidelity and the sense of copresence in all design elements, including physical layout, lighting, face tracking, multi-view capture, microphone array, multi-stream compression, loudspeaker output, and lenticular display. Our system achieves key 3D audiovisual cues (stereopsis, motion parallax, and spatialized audio) and enables the full range of communication cues (eye contact, hand gestures, and body language), yet does not require special glasses or body-worn microphones/headphones. The system consists of a head-tracked autostereoscopic display, high-resolution 3D capture and rendering subsystems, and network transmission using compressed color and depth video streams. Other contributions include a novel image-based geometry fusion algorithm, free-space dereverberation, and talker localization.
Author(s)/Presenter(s):
Jason Lawrence, Google Research, United States of America
Dan B. Goldman, Google Research, United States of America
Supreeth Achar, Google Research, United States of America
Gregory Major Blascovich, Google Research, United States of America
Joseph G. Desloge, Google Research, United States of America
Tommy Fortes, Google Research, United States of America
Eric M. Gomez, Google Research, United States of America
Sascha Häberling, Google Research, United States of America
Hugues Hoppe, Google Research, United States of America
Andy Huibers, Google Research, United States of America
Claude Knaus, Google Research, United States of America
Brian Kuschak, Google Research, United States of America
Ricardo Martin-Brualla, Google Research, United States of America
Harris Nover, Google Research, United States of America
Andrew I. Russell, NVIDIA, United States of America
Steven M. Seitz, Google Research, United States of America
Kevin Tong, Google Research, United States of America
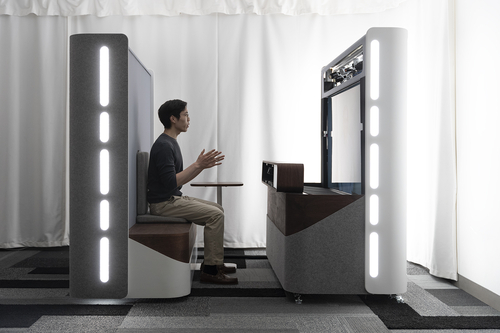
Reproducing Reality with a High-Dynamic-Range Multi-Focal Stereo Display
Abstract: With well-established methods for producing photo-realistic results, the next big challenge of graphics and display technologies is to achieve perceptual realism --- producing imagery indistinguishable from real-world 3D scenes. To deliver all necessary visual cues for perceptual realism, we built a High-Dynamic-Range Multi-Focal Stereo Display that achieves high resolution, accurate color, a wide dynamic range, and most depth cues, including binocular presentation and a range of focal depth. The display and associated imaging system have been designed to capture and reproduce a small near-eye three-dimensional object and to allow for a direct comparison between virtual and real scenes. To assess our reproduction of realism and demonstrate the capability of the display and imaging system, we conducted an experiment in which the participants were asked to discriminate between a virtual object and its physical counterpart. Our results indicate that the participants can only detect the discrepancy with a probability of 0.44. With such a level of perceptual realism, our display apparatus can facilitate a range of visual experiments that require the highest fidelity of reproduction while allowing for the full control of the displayed stimuli.
Author(s)/Presenter(s):
Fangcheng Zhong, University of Cambridge, United Kingdom
Akshay Jindal, University of Cambridge, United Kingdom
Ali Özgür Yöntem, University of Cambridge, United Kingdom
Param Hanji, University of Cambridge, United Kingdom
Simon Watt, Bangor University, United Kingdom
Rafal Mantiuk, University of Cambridge, United Kingdom
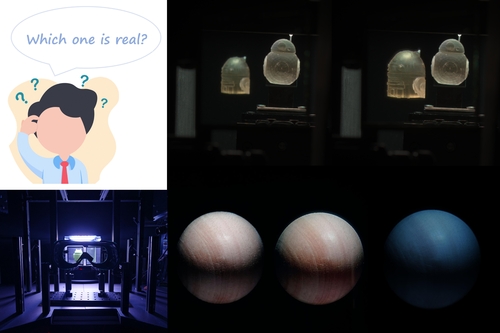
VR Social Copresence with Light Field Displays
Abstract: As virtual reality (VR) devices become increasingly commonplace, asymmetric interactions between people with and without headsets are becoming more frequent. Existing video pass-through VR headsets solve one side of these asymmetric interactions by showing the user a live reconstruction of the outside world. This paper further advocates for reverse pass-through VR, wherein a three-dimensional view of the user's face and eyes is presented to any number of outside viewers in a perspective-correct manner using a light field display. Tying together research in social telepresence and copresence, autostereoscopic displays, and facial capture, reverse pass-through VR enables natural eye contact and other important non-verbal cues in a wider range of interaction scenarios, providing a path to potentially increase the utility and social acceptability of VR headsets in shared and public spaces.
Author(s)/Presenter(s):
Nathan Matsuda, Facebook Reality Labs, United States of America
Brian Wheelwright, Facebook Reality Labs, United States of America
Joel Hegland, Facebook Reality Labs, United States of America
Douglas Lanman, Facebook Reality Labs, United States of America
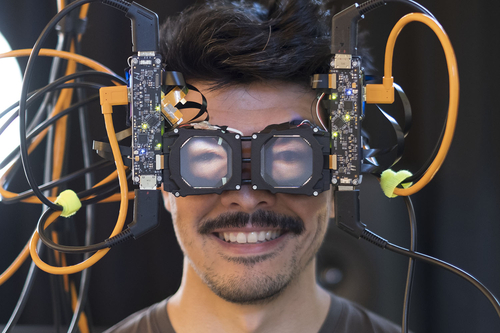