Machine Learning for Graphics [Q&A Session]
-
Full Access
-
Virtual Full Access
Date/Time: 06 – 17 December 2021
All presentations are available in the virtual platform on-demand.
A Multi-Stage Advanced Deep Learning Graphics Pipeline
Contributor(s):
Mark Wesley Harris, University of Colorado, Colorado Springs, United States of America
Sudhanshu Kumar Semwal, University of Colorado, Colorado Springs, United States of America
Description: We show how the Advanced Deep Learning Graphics Pipeline may be used to generate novel frames of simple 3D scenes, and could be applied to speed up current rendering processes.
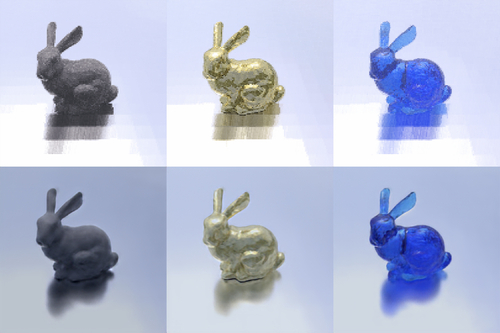
Anime Character Colorization using Few-shot Learning
Contributor(s):
Akinobu Maejima, OLM Digital, Inc.; IMAGICA GROUP, Inc., Japan
Hiroyuki Kubo, Tokai University, Japan
Seitaro Shinagawa, Nara Institute of Science and Technology (NAIST), Japan
Takuya Funatomi, Nara Institute of Science and Technology (NAIST), Japan
Tatsuo Yotsukura, OLM Digital, Inc.; IMAGICA GROUP, Inc., Japan
Satoshi Nakamura, Nara Institute of Science and Technology (NAIST), Japan
Yasuhiro Mukaigawa, Nara Institute of Science and Technology (NAIST), Japan
Description: We propose an automatic Anime-style colorization method using only a small number of colorized reference images manually colorized by artists. Our method helps to reduce the manual labor for artists.
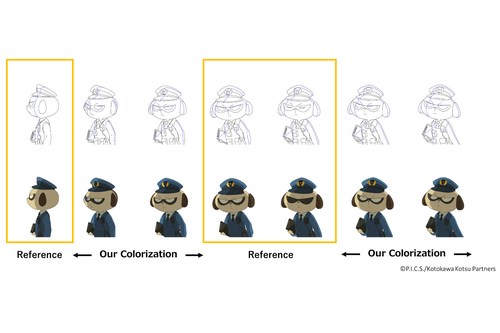
Comic Image Inpainting via Distance Transform
Contributor(s):
Naoki Ono, University of Tokyo, Japan
Kiyoharu Aizawa, University of Tokyo, Japan
Yusuke Matsui, University of Tokyo, Japan
Description: We propose a novel method of comic image inpainting. The conventional methods often fail to inpaint line drawings. We solve this problem by transforming line drawings into distance images.
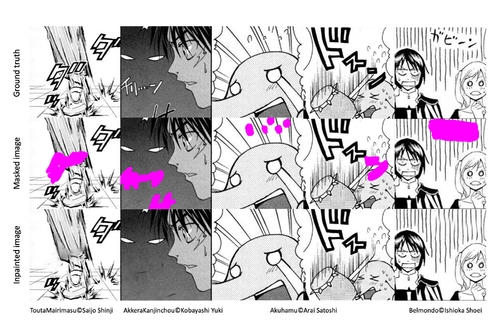
Guided Image Weathering using Image-to-Image Translation
Contributor(s):
Li-Yu Chen, National Taiwan University, Taiwan
I-Chao Shen, The University of Tokyo, Taiwan
Bing-Yu Chen, National Taiwan University, Taiwan
Description: We present a guided image weathering method. An image-to-image model is trained for transferring an age map to relative texture. New weathering results are generated by manipulating the age map.
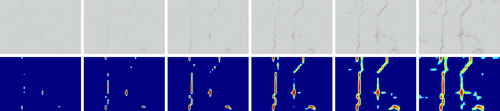